Email Spam Filter Machine Learning: A Game Changer for IT Services and Security Systems
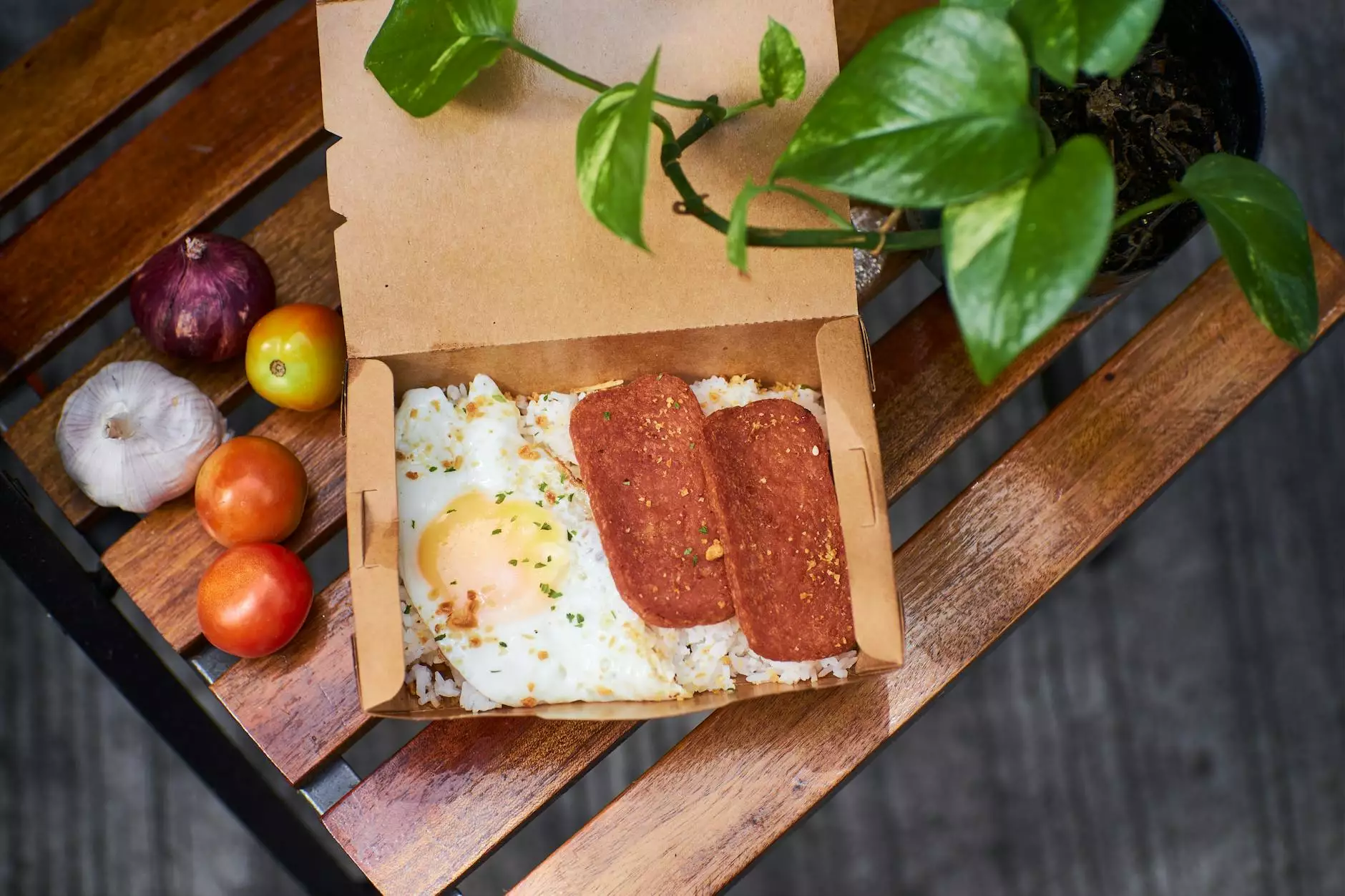
Introduction to Email Spam Filtering and Machine Learning
The digital landscape is constantly evolving, and so are the threats that businesses face every day. Among these threats, email spam stands out due to its prevalence and potential to disrupt operations. This is where email spam filter machine learning technologies come into play, serving as a frontline defense against unwanted and malicious emails.
Utilizing advanced machine learning algorithms, modern spam filters learn and adapt over time, significantly enhancing the security of organizations' IT services and computer systems. By understanding how these machine learning techniques operate, companies can improve their email security protocols, ultimately leading to a more efficient and secure business environment.
Understanding Email Spam and Its Impact on Businesses
Email spam refers to unsolicited and often irrelevant messages sent in bulk over email. These messages can have a variety of negative impacts on businesses, including:
- Wasted Resources: Processing and managing spam emails consumes significant bandwidth and time.
- Increased Vulnerability: Spam emails often contain malicious links or attachments, posing a serious security threat.
- Loss of Productivity: Employees may be distracted or overwhelmed by a flood of spam emails.
Organizations that do not employ effective spam filtering solutions can suffer from reputational damage and loss of sensitive information. This highlights the necessity of innovations like email spam filter machine learning in protecting business interests.
How Machine Learning Enhances Email Spam Filtering
Traditional spam filters used static rules to categorize emails. However, the evolving nature of spam tactics requires a more dynamic approach. Machine learning enables spam filters to analyze data and learn from it, leading to improved accuracy and effectiveness in filtering out unwanted emails.
Here’s how machine learning enhances email spam filtering:
- Data Processing: Machine learning algorithms analyze vast amounts of data to recognize patterns in spam and legitimate emails.
- Continuous Learning: As spam tactics evolve, these filters learn from new data, adapting to emerging threats.
- Improved Accuracy: Algorithms can achieve higher accuracy rates by minimizing false positives and false negatives.
The outcome is a significant enhancement in the quality of email filtering, offering a powerful solution to the spam problem faced by businesses today.
Core Techniques in Machine Learning for Spam Filtering
Various machine learning techniques contribute to effective email spam filtering. Below are some of the most prominent methods:
- Naive Bayes Classifier: Based on Bayes' theorem, this probabilistic model is often used for spam classification. It evaluates the likelihood of an email being spam based on the presence of certain words or phrases.
- Support Vector Machine (SVM): This technique finds the best boundary to separate spam from non-spam emails by identifying relevant features in the email content.
- Artificial Neural Networks (ANN): These systems mimic human neural networks to identify complex patterns in large datasets, providing high accuracy in classifying emails.
- Decision Trees: By utilizing a flowchart-like structure, decision trees analyze the features of emails and make decisions based on predefined criteria.
Choosing the right technique depends on the specific needs of the organization, the volume of emails processed, and the nature of the spam threats encountered.
Implementing Machine Learning-Based Spam Filters in IT Services
Integrating email spam filter machine learning into your IT services requires careful planning and execution. Here are the essential steps for implementation:
- Assess Organizational Needs: Understand the volume of incoming emails and the current challenges faced with spam filtering.
- Select the Right Tool: Choose a machine learning-based spam filtering solution that aligns with your specific requirements.
- Training the Model: Gather historical email data to train the machine learning model, helping it to recognize spam effectively.
- Continual Monitoring: Regularly evaluate the performance of the spam filter and make adjustments as new data is collected.
- User Feedback: Encourage employees to report spam that slips through the filter, enhancing the model’s accuracy over time.
By following these steps, businesses can successfully implement a robust spam filtering solution that enhances their overall security posture.
The Benefits of Using Machine Learning in Spam Filtering
Utilizing machine learning in spam filtering offers numerous advantages to organizations:
- Reduced Risk: Enhanced protection against phishing attacks and malware.
- Improved Efficiency: Filtering out irrelevant emails leads to improved productivity for employees.
- Cost Savings: Less time and resources spent on managing spam and related attacks can lead to significant cost savings over time.
- Better User Experience: A cleaner inbox enhances overall user satisfaction and engagement.
As cyber threats continue to evolve, businesses must adapt their defenses. Incorporating email spam filter machine learning not only addresses current challenges but also prepares organizations for future threats.
Future Trends in Email Spam Filtering and Machine Learning
The future of email spam filtering is bright, with continuous advancements in machine learning. Here are some trends to watch for:
- Integration with AI: The combination of AI and machine learning will further enhance spam detection capabilities.
- Contextual Filtering: Future filters will consider the context of emails to better differentiate between legitimate and spam emails.
- Real-time Threat Intelligence: Leveraging real-time data from global networks for immediate updates on emerging spam threats.
- Privacy-Preserving Techniques: As privacy concerns grow, developing machine learning methods that respect user privacy will become paramount.
By staying ahead of these trends, businesses can evolve their email security measures and maintain a robust defense against spam and cyber threats.
Conclusion: Securing Your Business with Email Spam Filter Machine Learning
In today's digital age, the importance of effective email spam filtering cannot be overstated. With the advent of email spam filter machine learning, businesses have the ability to significantly enhance their IT services and fortify their security systems against an increasing tide of spam threats.
Investing in machine learning-based spam filters is not merely a protective measure; it is a proactive step towards ensuring that your organization operates efficiently and securely. By understanding and implementing these advanced technologies, businesses can focus on growth and innovation without the looming threat of spam disrupting operations.
For more details on IT services and security systems, visit spambrella.com.